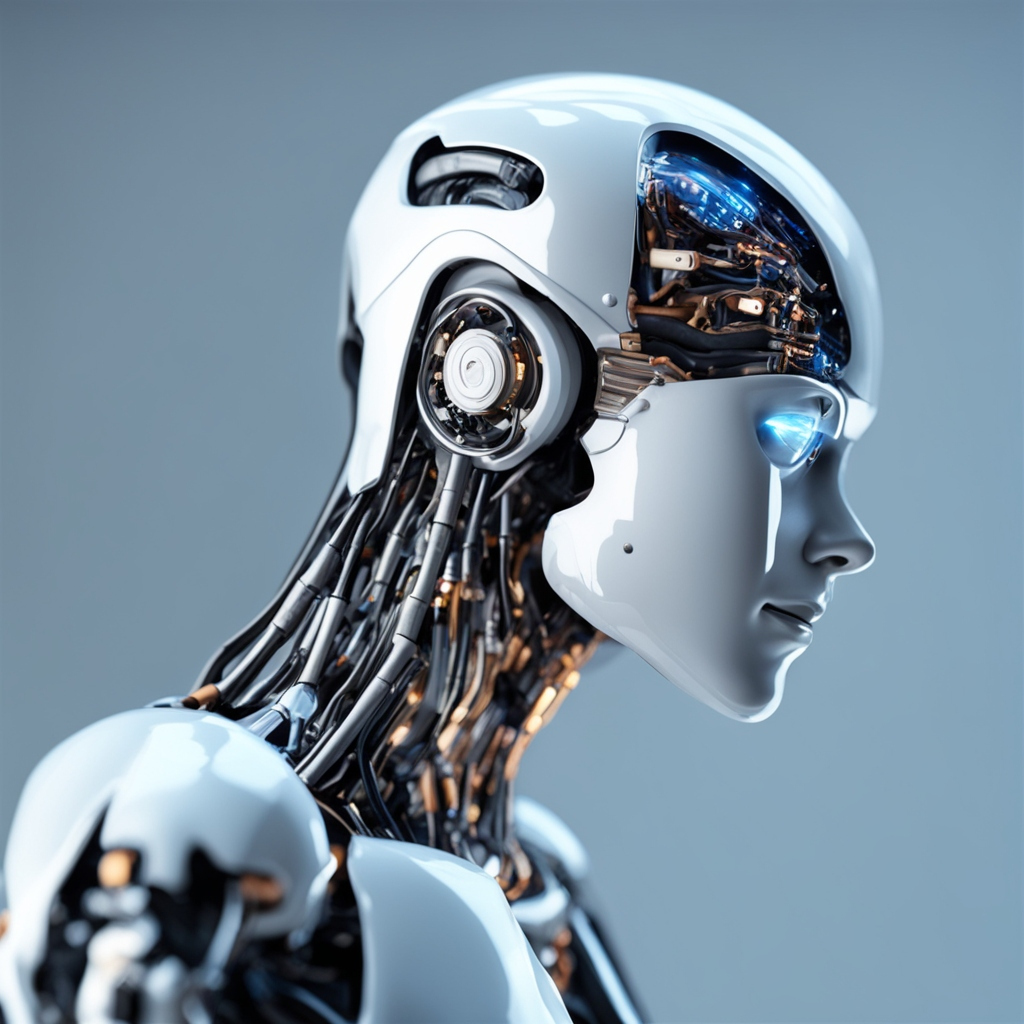
Artificial Intelligence (AI) and neural networks are at the heart of the technological revolution reshaping our world. From voice assistants and recommendation systems to autonomous vehicles and medical diagnostics, these technologies drive advancements across numerous domains. But how do AI and neural networks work? This article aims to provide a technical exploration of these concepts, breaking down their fundamental principles and mechanisms.
What is AI?
Understanding Artificial Intelligence
Artificial Intelligence refers to the simulation of human intelligence in machines. These systems are designed to perform tasks that typically require human cognitive functions such as learning, reasoning, problem-solving, perception, and language understanding. AI can be categorized into two main types:
- Narrow AI: Designed for specific tasks (e.g., virtual assistants like Siri).
- General AI: A hypothetical system capable of performing any intellectual task that a human can do.
The Core Components of AI
AI systems consist of several core components:
- Data: The foundational element that fuels AI. Data is collected, processed, and analyzed to train models.
- Algorithms: Mathematical formulas and procedures that define the step-by-step operations of the AI system.
- Models: Structures built through training algorithms on data, enabling the AI system to make predictions or decisions.
- Computing Power: The hardware and infrastructure required to process large datasets and complex algorithms efficiently.
The Role of Neural Networks
Introduction to Neural Networks
Neural networks are a subset of AI and are inspired by the structure and function of the human brain. They consist of interconnected nodes, or neurons, organized into layers. These networks are capable of learning patterns from data and making decisions based on those patterns.
Structure of a Neural Network
A neural network typically comprises three types of layers:
- Input Layer: Receives the initial data.
- Hidden Layers: Intermediate layers where the actual computation and learning occur. There can be multiple hidden layers, making the network deep (hence the term “deep learning”).
- Output Layer: Produces the final result or prediction.
Each node in a layer is connected to nodes in the next layer through weighted connections. The weights are adjusted during the training process to minimize the error in the network’s predictions.
Types of Neural Networks
Different types of neural networks are designed for specific tasks:
- Feedforward Neural Networks (FNN): The simplest type where data moves in one direction from input to output.
- Convolutional Neural Networks (CNN): Specialized for image processing and computer vision tasks, using convolutional layers to detect spatial hierarchies.
- Recurrent Neural Networks (RNN): Suitable for sequential data such as time series or natural language, with feedback loops that allow information to persist.
- Generative Adversarial Networks (GAN): Comprising two networks, a generator and a discriminator, that work against each other to create realistic synthetic data.
Training Neural Networks
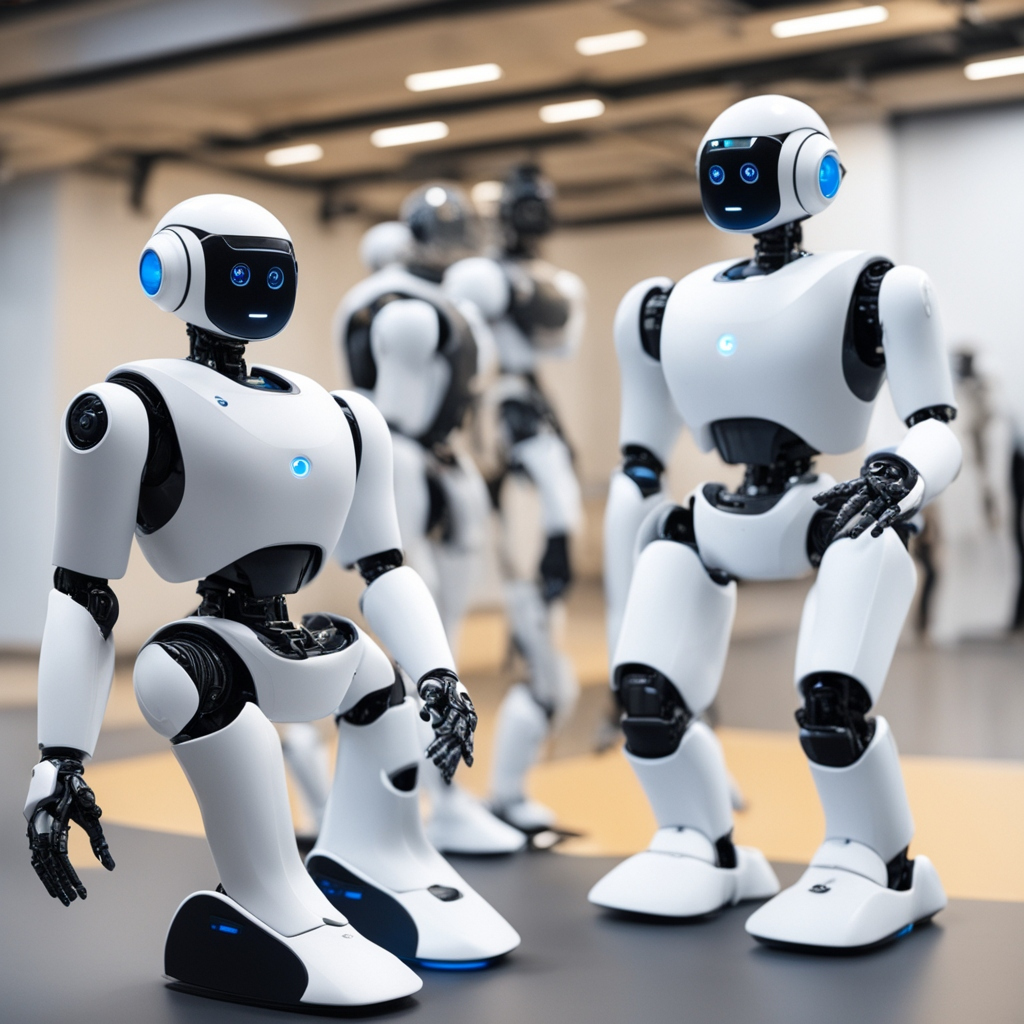
The Training Process
Training a neural network involves adjusting the weights of connections to minimize the difference between the predicted output and the actual output. This process typically includes the following steps:
- Forward Propagation: Data is passed through the network, producing an output.
- Loss Calculation: The error (loss) between the predicted output and the actual output is calculated using a loss function.
- Backward Propagation (Backpropagation): The network adjusts the weights of the connections based on the error gradient. This is done using optimization algorithms like Gradient Descent.
- Iteration: The process is repeated for multiple epochs (full passes through the training dataset) until the model achieves a desired level of accuracy.
Activation Functions
Activation functions introduce non-linearity into the network, enabling it to learn complex patterns. Common activation functions include:
- Sigmoid: Produces an output between 0 and 1, often used in binary classification.
- ReLU (Rectified Linear Unit): Outputs zero if the input is negative; otherwise, it outputs the input itself, helping mitigate the vanishing gradient problem.
- Tanh: Outputs values between -1 and 1, often used in layers where the mean of the activations should be close to zero.
Overfitting and Regularization
Overfitting occurs when a model learns the training data too well, including noise and outliers, which reduces its generalization to new data. Regularization techniques such as dropout, L1/L2 regularization, and early stopping are employed to prevent overfitting and improve model generalization.
Applications of AI and Neural Networks
Computer Vision
Neural networks, particularly CNNs, are extensively used in computer vision tasks like image classification, object detection, and facial recognition. These models can analyze and interpret visual data with high accuracy.
Natural Language Processing (NLP)
RNNs and transformers are pivotal in NLP tasks, including language translation, sentiment analysis, and chatbots. These networks can understand and generate human language, making them integral to applications like virtual assistants and automated customer support.
Healthcare
AI and neural networks are revolutionizing healthcare by enabling early disease detection, personalized treatment plans, and predictive analytics. Models trained on medical data can assist in diagnosing conditions from medical images or predicting patient outcomes.
Autonomous Systems
Self-driving cars, drones, and robots rely on neural networks to perceive their environment, make decisions, and navigate safely. These systems integrate data from various sensors and use AI to operate autonomously.
Artificial Intelligence and neural networks are transforming industries and reshaping our daily lives. By mimicking the learning processes of the human brain, these technologies can analyze vast amounts of data, recognize patterns, and make informed decisions. As research and development in AI continue to advance, we can expect even more innovative applications and breakthroughs that will further integrate these systems into our world.
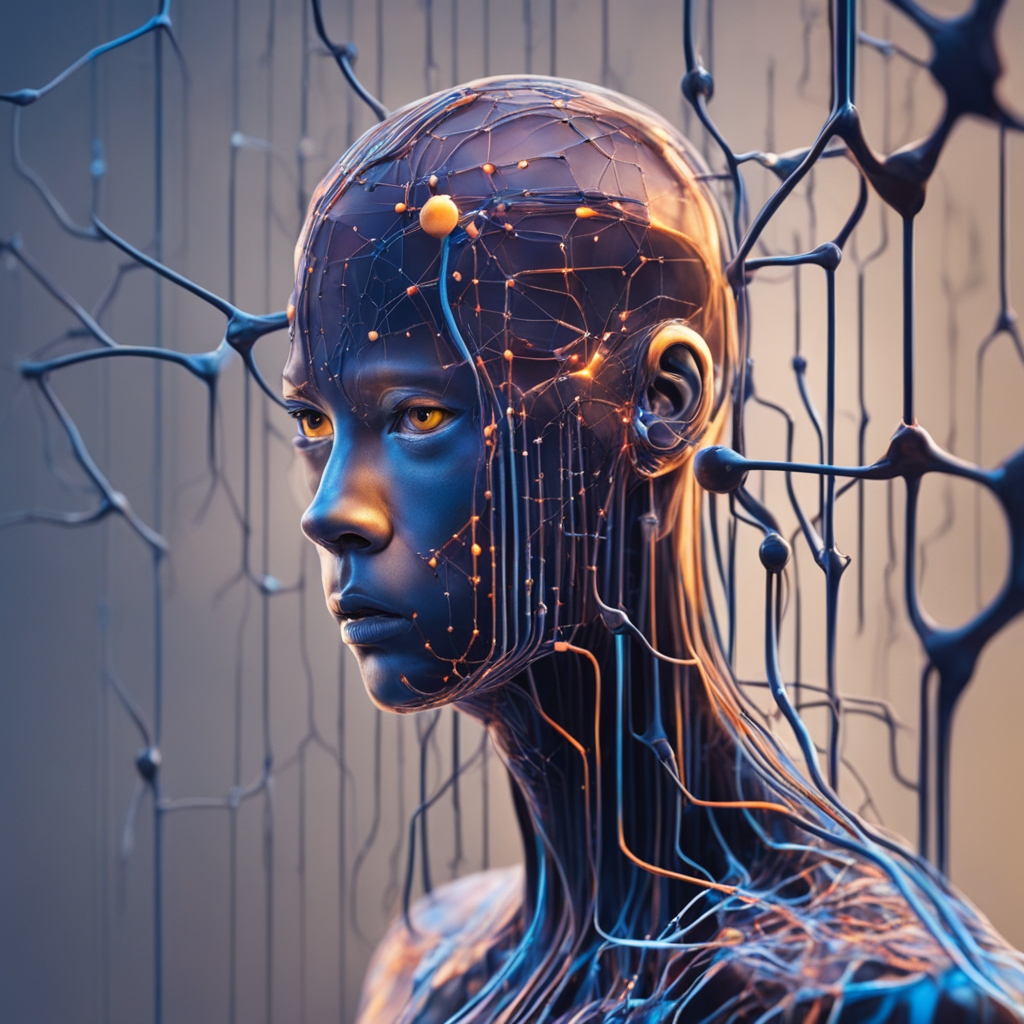
Understanding the technical foundations of AI and neural networks not only demystifies their workings but also highlights their immense potential. From enhancing productivity and efficiency to unlocking new frontiers of knowledge and capability, AI and neural networks are truly the engines driving the future of technology.